Lidar and Monocular Sensor Fusion Depth Estimation
DOI:
https://doi.org/10.5281/zenodo.11347309Keywords:
lidar, fusion, monocular sensorAbstract
In this project, we present a novel approach to depth perception using a monocular camera by incorporating information from both RGB and LiDAR modalities. Our primary objective is to investigate the performance and effectiveness of different techniques to generate accurate depth estimation. We first implemented the Swin Transformer-based depth estimation model and evaluated its performance on KITTI dataset containing RGB images and their corresponding ground truth depth maps. Next, we proposed an RGB-LiDAR fusion model. We performed necessary preprocessing steps on the dataset, such as resizing, normalization, and data augmentation, and trained both models with identical configurations for a fair comparison. Our results demonstrate that the proposed RGB- LiDAR fusion model achieves superior depth estimation performance compared to the original Swin Transformer based model. We evaluated the models on the test dataset using metrics such as mean absolute error (MAE) and root mean squared error (RMSE). The enhanced performance indicates the potential benefits of RGB-LiDAR fusion for monocular depth perception tasks. This study offers valuable insights into the strengths [1] and weaknesses of combining RGB and LiDAR inputs and lays the foundation for future research in monocular depth perception, aiming to further improve model architectures and training techniques.
Downloads
References
J. Jin, F. Ni, S. Dai, K. Li, & B. Hong. (2024). Enhancing federated semi-supervised learning with out-of-distribution filtering amidst class mismatches. Journal of Computer Technology and Applied Mathematics, 1(1), 100–108.
S. Li, Y. Mo, & Z. Li. (2022). Automated pneumonia detection in chest x-ray images using deep learning model. Innovations in Applied Engineering and Technology, 1–6.
Z. Li, H. Yu, J. Xu, J. Liu, & Y. Mo. (2023). Stock market analysis and prediction using lstm: A case study on technology stocks. Innovations in Applied Engineering and Technology, 1–6.
K. Li, P. Xirui, J. Song, B. Hong, & J. Wang. (2024). The application of augmented reality (ar) in remote work and education. arXiv preprint arXiv:2404.10579.
K. Li, A. Zhu, P. Zhao, J. Song, & J. Liu. (2024). Utilizing deep learning to optimize software development processes. Journal of Computer Technology and Applied Mathematics, 1(1), 70–76.
T. Lin, & J. Cao. (2020). Touch interactive system design with intelligent vase of psychotherapy for alzheimer’s disease. Designs, 4(3), 28.
T. Liu, S. Li, Y. Dong, Y. Mo, & S. He. (2024). Spam detection and classification based on distilbert deep learning algorithm. Applied Science and Engineering Journal for Advanced Research, 3(3), 6–10.
Y. Mo, H. Qin, Y. Dong, Z. Zhu, & Z. Li. (2024). Large language model (llm) ai text generation detection based on transformer deep learning algorithm. International Journal of Engineering and Management Research, 14(2), 154–159.
A. Xiang, J. Zhang, Q. Yang, L. Wang, & Y. Cheng. (2024). Research on splicing image detection algorithms based on natural image statistical characteristics. arXiv preprint arXiv:2404.16296.
Y. Mo, S. Li, Y. Dong, Z. Zhu, & Z. Li. (2024). Password complexity prediction based on roberta algorithm. Applied Science and Engineering Journal for Advanced Research, 3(3), 1–5.
J. Cao, D. Ku, J. Du, V. Ng, Y. Wang, & W. Dong. (2017). A structurally enhanced, ergonomically and human–computer interaction improved intelligent seat’s system. Designs, 1(2), 11.
H. Jiang, F. Qin, J. Cao, Y. Peng, & Y. Shao. (2021). Recurrent neural network from adder’s perspective: Carry-lookahead rnn. Neural Networks, 144, 297–306.
P. Mu, W. Zhang, & Y. Mo. (2021). Research on spatio-temporal patterns of traffic operation index hotspots based on big data mining technology. in Basic & Clinical Pharmacology & Toxicology, 128, Wiley 111 River St, Hoboken 07030-5774, NJ USA, pp. 185–185.
J. Zhang, A. Xiang, Y. Cheng, Q. Yang, & L. Wang. (2024). Research on detection of floating objects in river and lake based on ai intelligent image recognition. arXiv preprint arXiv:2404.06883.
J. Song, H. Liu, K. Li, J. Tian, & Y. Mo. (2024). A comprehensive evaluation and comparison of enhanced learning methods. Academic Journal of Science and Technology, 10(3), 167–171.
A. Zhu, K. Li, T. Wu, P. Zhao, & B. Hong. (2024). Cross-task multi-branch vision transformer for facial expression and mask wearing classification. Journal of Computer Technology and Applied Mathematics, 1(1), 46–53.
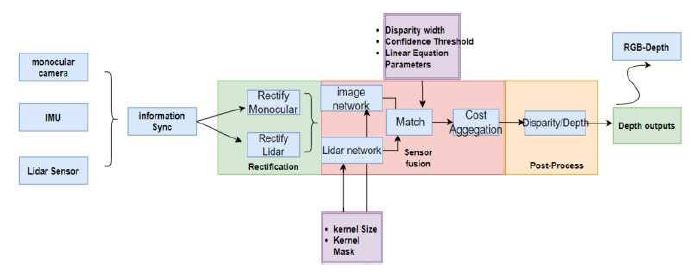
Downloads
Published
How to Cite
Issue
Section
ARK
License
Copyright (c) 2024 Shuyao He, Yue Zhu, Yushan Dong, Hao Qin, Yuhong Mo

This work is licensed under a Creative Commons Attribution 4.0 International License.
Research Articles in 'Applied Science and Engineering Journal for Advanced Research' are Open Access articles published under the Creative Commons CC BY License Creative Commons Attribution 4.0 International License http://creativecommons.org/licenses/by/4.0/. This license allows you to share – copy and redistribute the material in any medium or format. Adapt – remix, transform, and build upon the material for any purpose, even commercially.