Precision in Practice: Enhancing Healthcare with Domain-Specific Language Models
DOI:
https://doi.org/10.5281/zenodo.13253336Keywords:
domain-specific language models (dslms), medical information dissemination, telehealth integrationAbstract
This paper investigates the role of domain-specific language models (DSLMs) in enhancing the accuracy and reliability of responses to general medical inquiries. Focusing on the healthcare sector, we explore how LLMs, when fine-tuned with specialized medical datasets, surpass general language models in handling complex medical terminology and ensuring patient data confidentiality. The benefits of these models include increased precision in medical advice, a reduction in misinformation risks, and tailored responses based on individual patient histories. Nonetheless, implementing these technologies comes with significant ethical, technical, and regulatory challenges, such as ensuring patient privacy, maintaining up-to-date medical knowledge, and navigating stringent compliance requirements. The paper concludes by discussing future directions, including the integration of DSLMs with telehealth services and ongoing advancements in model training techniques, underscoring the necessity for continued research, widespread adoption, and rigorous evaluation to fully leverage their potential in improving healthcare outcomes.
Downloads
References
Li, S., Mo, Y., & Li, Z. (2022). Automated pneumonia detection in chest x-ray images using deep learning model. Innovations in Applied Engineering and Technology, 1(1), 1–6.
Haoran Yu, Chang Yu, Zihan Wang, Dongxian Zou, & Hao Qin. (2024). Enhancing healthcare through large language models: A study on medical questions answering. Proceedings to 2024 IEEE 6th International Conference on Power, Intelligent Computing and Systems (ICPICS).
Li, S., Mo, Y., & Li, Z. (2022). Automated pneumonia detection in chest x-ray images using deep learning model. Innovations in Applied Engineering and Technology, 1(1), 1–6.
Dang, Bo, et al. (2024). Real-Time pill identification for the visually impaired using deep learning. arXiv preprintarXiv:2405.05983.
Hao Qin, & Zhi Li. (2024). A study on enhancing government efficiency and public trust: The transformative role of artificial intelligence and large language models. International Journal of Engineering and Management Research, 14(3), 57–61. https://doi.org/10.5281/zenodo.12619360.
Li, Z. ., Yu, H., Xu, J., Liu, J., & Mo, Y. (2023). Stock market analysis and prediction using LSTM: A case study on technology stocks. Innovations in Applied Engineering and Technology, 2(1), 1–6.
Hao Qin. (2024). Revolutionizing cryptocurrency operations: The role of domain-specific large language models (LLMs). International Journal of Computer Trends and Technology, 72(6), 101-113.
Dai, S., Dai, J., Zhong, Y., Zuo, T., & Mo, Y. (2024). The cloud-based design of unmanned constant temperature food delivery trolley in the context of artificial intelligence. Journal of Computer Technology and Applied Mathematics, 1(1), 6–12.
Ma, Danqing, et al. (2024). Fostc3net: A lightweight yolov5 based on the network structure optimization. arXiv preprint arXiv:2403.13703.
Yuhong Mo, Chaoyi Tan, Chenghao Wang, Hao Qin, & Yushan Dong. (2024). Make scale invariant feature transform “Fly” with CUDA. International Journal of Engineering and Management Research, 14(3), 38–45. https://doi.org/10.5281/zenodo.11516606.
Shuyao He, Yue Zhu, Yushan Dong, Hao Qin, & Yuhong Mo. (2024). Lidar and monocular sensor fusion depth estimation. Applied Science and Engineering Journal for Advanced Research, 3(3), 20–26. https://doi.org/10.5281/zenodo.11347309.
Shaojie Li, Xinqi Dong, Danqing Ma, Bo Dang, Hengyi Zang, & Yulu Gong. Utilizing the light gbm algorithm for operator user credit assessment research, Applied and Computational Engineering, 75, 36-47.
Zeyu Wang, Yue Zhu, Zichao Li, Zhuoyue Wang, Hao Qin, & Xinqi Liu. (2024). Graph neural network recommendation system for football formation. Applied Science and Biotechnology Journal for Advanced Research, 3(3), 33–39. https://doi.org/10.5281/zenodo.12198843.
Liu, Xiaoyi, & Zhuoyue Wang. (2024). Deep learning in medical image classification from mri-based brain tumor images. arXiv preprint arXiv:2408.00636.
Mo, Y., Qin, H., Dong, Y., Zhu, Z., & Li, Z. (2024). Large Language Model (LLM) AI text generation detection based on transformer deep learning algorithm. International Journal of Engineering and Management Research, 14(2), 154–159. https://doi.org/10.5281/zenodo.11124440.
Zheng Lin, Zeyu Wang, Yue Zhu, Zichao Li, & Hao Qin. (2024). Text sentiment detection and classification based on integrated learning algorithm. Applied Science and Engineering Journal for Advanced Research, 3(3), 27–33. https://doi.org/10.5281/zenodo.11516191.
Bo Dang, Danqing Ma, Shaojie Li, Zongqing Qi, & Elly Zhu. (2024). Deep learning-based snore sound analysis for the detection of night-time breathing disorders. Applied and Computational Engineering, 76, 109–114.
Zhi Li. (2024). Ethical frontiers in artificial intelligence: navigating the complexities of bias, privacy, and accountability. International Journal of Engineering and Management Research, 14(3), 109–116. https://doi.org/10.5281/zenodo.12792741.
Zhao, W., Liu, X., Xu, R., Xiao, L., & Li, M. (2024). E-commerce webpage recommendation scheme base on semantic mining and neural networks. Journal of Theory and Practice of Engineering Science, 4(03), 207–215. https://doi.org/10.53469/jtpes.2024.04(03).20.
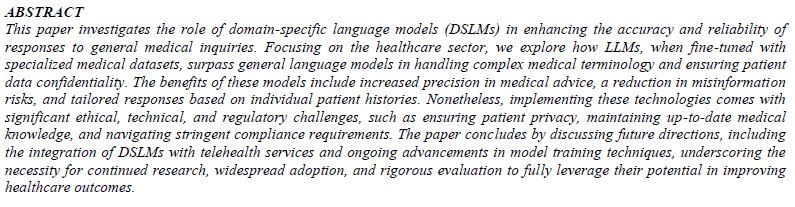
Downloads
Published
How to Cite
Issue
Section
License
Copyright (c) 2024 Hao Qin, Zhi Li

This work is licensed under a Creative Commons Attribution 4.0 International License.