Decision Support Model in Production and Customer Networks
DOI:
https://doi.org/10.54741/asejar.2.4.6Keywords:
industrial, service networks, model based, decision maker, challenges, productAbstract
We outline some of the issues that need to be taken into consideration in upcoming studies on model-based decision support in service networks and manufacturing. Integration problems that look at how independent the decision-making entities are when there is an imbalance of information, decision-maker preference modeling, finding robust solutions (solutions that don't change when the problem data changes), and shortening the time it takes to make and use models are all covered. The process of solving a problem involves analyzing the problem, designing suitable algorithms, and evaluating how well those algorithms work. We are interested in a field test using the expanded application systems after a prototype integration of the suggested ways within application systems. We contend that the proposed research agenda necessitates the interdisciplinary cooperation of researchers in business and information systems engineering with associates in computer science, management science, and operations research. We also provide a few representative examples of pertinent research findings.
Downloads
References
Rose, O. (2007). Improved simple simulation models for semiconductor wafer fabrication facilities. in Proc of the 2007 Winter Simulation Conference, 1708–1712.
Graves SC. (2010). Uncertainty and production planning. Heidelberg, pp. 83-102.
Bilyk A, & Mönch L. (2012). Variable neighborhood search-based subproblem solution procedures for a parallel shifting bottleneck heuristic for complex job shops. in Proc of IEEE Conference on Automation Science and Engineering, Seoul, pp. 415–420.
Rothlauf F. (2011). Design of modern heuristics: Principles and application. Springer, Heidelberg.
Schütte R. (2012). In-memory-technologien: Überlegungen zur Begründbarkeit und zum Einsatz beim Betrieb von großen Systemen, WI-Meinung/Dialog. Wirtschafts Informatik 54(4), 211–213.
Nisan, N, Roughgarden, T, Tardos, E, & Vazirani, VV. (2007). Algorithmic game theory. New York: Cambridge University Pres.
Abbasi, S. Sarker, & R. Chiang. (2016). Big data research in information systems: Toward an inclusive research agenda. Journal of the Association for Information Systems, 17(2), 3.
G. Andreadis, P. Klazoglou, K. Niotaki, & K.-D. Bouzakis.(2014).Classification and review of multi-agents systems in the manufacturing section. Procedia Engineering, 69, 282–290.
C. J. Hanlon, J. B. Stefik, A. A. Small, J. Verlinde. (2013). Young statistical decision analysis for flight decision support: The sparticus campaign. Journal of Geophysical Research: Atmospheres, 118(10), 4679–4688.
R. de FSM, & Russo & R. (2015). Camanho. criteria in AHP: A systematic review of literature. Procedia Computer Science, 55, 1123–1132.
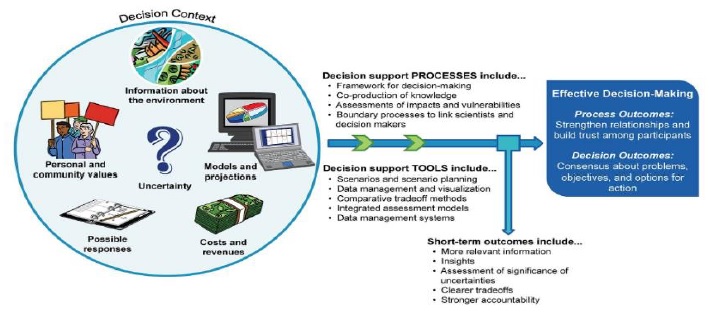
Downloads
Published
How to Cite
Issue
Section
ARK
License
Copyright (c) 2023 Dr. Mukesh Mishra

This work is licensed under a Creative Commons Attribution 4.0 International License.
Research Articles in 'Applied Science and Engineering Journal for Advanced Research' are Open Access articles published under the Creative Commons CC BY License Creative Commons Attribution 4.0 International License http://creativecommons.org/licenses/by/4.0/. This license allows you to share – copy and redistribute the material in any medium or format. Adapt – remix, transform, and build upon the material for any purpose, even commercially.