Big Data and Computer-Human Interaction for Real-Time Illness Diagnosis
DOI:
https://doi.org/10.5281/zenodo.10578916Keywords:
healthcare, stream processing, human computer interaction, big data, apache spark, distributed machine learning, internet of thingsAbstract
One particularly significant technology for deterrence of many chronic diseases is the constant plus real time tracking system, which is made possible through IoT and human computer interaction. Big data streaming stays the enormous volume of data that wearable medical procedures with sensors, healthcare clouds as well as mobile applications constantly produce. The increased pace of data collecting makes it challenging to gather, process as well as analyses such massive data sets in actual time in order to respond quickly in an emergency situation and unearth the hidden value. To offer an effective and scalable solution, real-time large data stream processing is therefore significantly needed. This work suggests a novel architecture for a big data based real time health prestige prediction as well as analytics system to address this problem. The system focuses on using a disseminated ML model to analyze health data events that are streamed into Spark via Kafka topics. First, we replace Hadoop MapReduce with Spark to produce a parallel, distributed, scalable, and rapid decision tree algorithm, which develops constrained for the real time computation. Second, this model is utilized to stream data from various sources that deal with numerous diseases in order to forecast health status. It is used to forecast health status using streaming data commencing distributed sources that represent various disorders.
Downloads
References
Manogaran G, & Lopez D. (2018). Health data analytics using scalable logistic regression with stochastic gradient descent. Int J Adv Intell Paradigms, 10(1–2), 118–32.
Hu H, Wen Y, Chua T‑S, & Li X. (2014). Toward scalable systems for big data analytics: A technology tutorial. IEEE Access, 2, 652–87.
Cattell R. (2011). Scalable sql and NoSQL data stores. ACM Sigmod Record, 39(4), 12–27.
Moniruzzaman A, & Hossain SA. (2013). NoSQL database: New era of databases for big data analytics‑classification, char‑ acteristics and comparison. arXiv preprint arXiv:1307.0191.
Dean J, & Ghemawat S. Mapreduce. (2008). Simplified data processing on large clusters. Commun ACM, 51(1), 107–13.
Belle A, Thiagarajan R, Soroushmehr S, Navidi F, Beard DA, & Najarian K. (2015). Big data analytics in healthcare. BioMed Res Int.
Bauer H, Patel M, & Veira J. (2017). The internet of things: Sizing up the opportunity. Available at: http://www.mckinsey.com/.
Zaharia M, Chowdhury M, Das T, Dave A, Ma J, McCauley M, Franklin MJ, Shenker S, & Stoica I. (2012). Resilient distributed datasets: A fault‑tolerant abstraction for in‑memory cluster computing. Proceedings of the 9th USENIX Conference on Networked Systems Design and Implementation.
https://www.kaggle.com/fmendes/diabetes‑from‑dat263x‑lab01.
Quinlan JR. (2014). C4. 5: Programs for machine learning. Amsterdam: Elsevier.
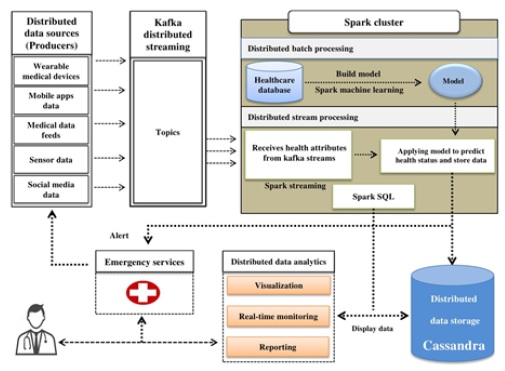
Downloads
Published
How to Cite
Issue
Section
ARK
License
Copyright (c) 2024 Sonam Goyal

This work is licensed under a Creative Commons Attribution 4.0 International License.
Research Articles in 'Applied Science and Engineering Journal for Advanced Research' are Open Access articles published under the Creative Commons CC BY License Creative Commons Attribution 4.0 International License http://creativecommons.org/licenses/by/4.0/. This license allows you to share – copy and redistribute the material in any medium or format. Adapt – remix, transform, and build upon the material for any purpose, even commercially.