Development in Deep Convolutional Neural Networks by using Machine Learning Framework
DOI:
https://doi.org/10.54741/asejar.2.2.2Keywords:
deep learning, neural networks, convolutional neural networksAbstract
In recent years, the machine learning technology has drawn more interest in a variety of vision tasks such image classification, image detection, and picture identification. Recent improvements in machine learning methods, in particular, stimulate the use of convolutional neural networks for image classification. CNNs are recognised as a potent class of models for image identification issues, sometimes even outperforming humans. The study described in this paper's major objective is to provide an overview of the rise and development of machine learning, deep learning, CNN, and the use of machine learning for image categorization. The CNN and conventional methods are contrasted at the conclusion.
Downloads
References
Yann LeCun, Yoshua Bengio, & Geoffrey Hinton. (2015). Deep learning. Nature, 521(1), 436-444.
Geoffrey E. Hinton, Simon Osindero, & Yee-Whye Teh. (2006). A fast learning algorithm for deep belief nets. Neural Computation, 18(1), 1527–1554.
Li Deng, & Dong Yu. (2013). Deep learning methods and applications. Foundations and Trends in Signal Processing, 7(3-4), 197-387.
Tom M. Mitchell. (2006). The discipline of machine learning. CMU-ML, 06(1), 108.
Rich Caruana, & Alexandru Niculescu-Mizil. (2006). An empirical comparison of supervised learning algorithms. International Conference on Machine Learning, 23(1), pp. 1-8.
Alex Krizhevsky, & Georey E. Hinton. (2011). Using very deep autoencoders for content-based image retrieval. University of Toronto - Department of Computer Science, 2(18), 34-56.
Waseem Rawat & Zenghui Wang. (2017). Deep convolutional neural networks for image classification; A comprehensive review.
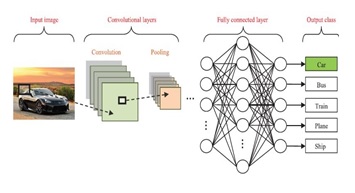
Published
How to Cite
Issue
Section
License
Copyright (c) 2023 Yousuf Tarbez

This work is licensed under a Creative Commons Attribution 4.0 International License.