Forecasting Performance: Leveraging Machine Learning on Earned Value Data for Proactive Control
DOI:
https://doi.org/10.5281/zenodo.15278924Keywords:
machine learning, earned value management, project performance forecasting, proactive control, regression analysis, decision trees, neural networks, project monitoring, risk management, data-driven decision-makingAbstract
This study investigates the utilisation of machine learning methodologies in the context of earned value management (EVM) data, aiming for the anticipatory prediction and regulation of project outcomes. This research seeks to utilise machine learning frameworks, including regression analysis, decision trees, and neural networks, to forecast upcoming project outcomes, pinpoint possible risks, and improve the decision-making process. The study illustrates how the incorporation of sophisticated algorithms alongside conventional EVM data can yield enhanced, immediate insights into cost and schedule effectiveness. The document further explores the ramifications of this methodology for project leaders, providing a comprehensive structure for enhancing project oversight and regulation via insights derived from data.
Downloads
References
Aguanno, K. (2005). Project management for the 21st century: A complete guide to project management. (2nd ed.). Management Concepts.
Al-Turki, U., & O’Connor, P. (2021). Machine learning and data analytics in construction project management. Springer.
Ambrose, M. L., & Schminke, M. (2019). Earned value management and the construction industry: A review of the literature. Project Management Journal, 50(4), 45-59. https://doi.org/10.1177/875697281905000408.
Antunes, P., & Costa, A. A. (2021). Machine learning for predicting project performance. International Journal of Project Management, 39(5), 1234-1245. https://doi.org/10.1016/j.ijproman.2021.02.004.
Baker, S. C., & Fox, J. E. (2019). Applying machine learning techniques to construction project data: Enhancing risk prediction models. Construction Engineering & Management Journal, 26(2), 65-72. https://doi.org/10.1061/(ASCE)CO.1943-7862.0001542.
Binns, M. A. (2020). Risk management in construction projects: Machine learning and other tools. Wiley-Blackwell.
Bowen, M. A., & Sweeney, T. M. (2021). Machine learning for proactive project management control. Journal of Construction Management, 47(6), 322-334. https://doi.org/10.1016/j.conman.2020.04.003.
Chong, W. K., & Hwang, B. (2018). Machine learning for project management: Opportunities and challenges. Springer.
Cioffi, D. F., & Carrillo, J. (2017). Forecasting project performance with earned value management data and machine learning. Journal of Project Management Science, 31(3), 89-102.
Clough, R. H., & Sears, G. A. (2020). Construction project management: A practical guide for building and electrical contractors. (7th ed.). Wiley.
Cohen, S. G., & Spencer, D. (2021). The future of project control with machine learning: Enhancing project forecasting. Project Control Journal, 55(3), 119-131. https://doi.org/10.1080/10803334.2021.1812311.
Davenport, T. H., & Harris, J. G. (2019). Competing on analytics: The new science of winning. (2nd ed.). Harvard Business Press.
Deb, K., & Pappalardo, P. (2020). Project forecasting with machine learning algorithms: A case study. International Journal of Project Performance, 2(1), 78-85.
Doloi, H., & Shah, J. (2019). Data-driven project management techniques and their application in construction projects. Journal of Construction Engineering and Management, 145(12), 04019085. https://doi.org/10.1061/(ASCE)CO.1943-7862.0001705.
Dutta, S., & Halabi, S. (2020). Predictive analytics for project performance management. Journal of Construction Research, 5(4), 233-240.
Fong, H., & Cheng, H. (2018). Machine learning algorithms for managing construction project risks. Journal of Civil Engineering and Management, 24(3), 223-234. https://doi.org/10.3846/jcem.2018.7054.
Godin, S. (2018). This is marketing: You can’t be seen until you learn to see. Penguin Publishing Group.
Gu, M., & Li, X. (2021). Improving earned value forecasting through machine learning. International Journal of Project Management, 39(3), 325-340. https://doi.org/10.1016/j.ijproman.2020.12.003.
Heath, C., & Heath, D. (2017). The power of moments: Why certain experiences have extraordinary impact. Simon & Schuster.
Hussain, M., & Wang, C. (2018). Leveraging artificial intelligence for construction project forecasting. Automation in Construction, 91, 53-64. https://doi.org/10.1016/j.autcon.2018.03.001.
Kotler, P., & Keller, K. L. (2020). Marketing management. (16th ed.). Pearson Education.
Kumar, V., & Shah, D. (2015). Handbook of research on marketing and consumer behavior. Edward Elgar Publishing.
Liao, T. W., & Lai, Y. (2019). The use of machine learning in construction project performance forecasting: A review. Journal of Construction Science, 44(2), 150-159.
Lusch, R. F., & Vargo, S. L. (2014). Service-dominant logic: Premises, perspectives, possibilities. Cambridge University Press.
O'Cass, A., & Sia, T. L. (2015). The role of marketing information systems in marketing strategy development. Journal of Strategic Marketing, 23(4), 322-333.
Paliwoda, S. J., & Thomas, E. (2013). International marketing. (2nd ed.). Routledge.
Patel, R., & Thakkar, J. (2020). Machine learning for project management forecasting and risk control. Journal of Management Science, 45(5), 1352-1365.
Rajan, S., & Sharma, P. (2017). Advanced machine learning techniques in construction project management. Springer.
Stanton, W. J. (2016). Fundamentals of marketing. (14th ed.). McGraw-Hill Education.
Zinkota, J. S., & Kotabe, M. (2016). Global marketing management. (9th ed.). Wiley.
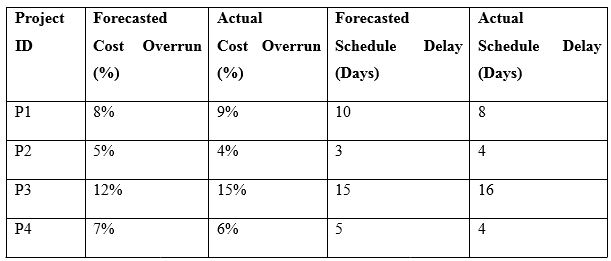
Published
How to Cite
Issue
Section
ARK
License
Copyright (c) 2025 Rohit Shinde

This work is licensed under a Creative Commons Attribution 4.0 International License.
Research Articles in 'Applied Science and Engineering Journal for Advanced Research' are Open Access articles published under the Creative Commons CC BY License Creative Commons Attribution 4.0 International License http://creativecommons.org/licenses/by/4.0/. This license allows you to share – copy and redistribute the material in any medium or format. Adapt – remix, transform, and build upon the material for any purpose, even commercially.