Beef Quality Classification and Logistics Patterns over Local, Regional, and National Supply Chains in South Korea
DOI:
https://doi.org/10.5281/zenodo.14835719Keywords:
machine learning, beef quality, decision tree, classification, supply chainAbstract
In this paper, we intend to examine the impact of several biological factors such as cattle types, gender, and weights on beef quality using data sets obtained from the digital beef traceability system in South Korea. In addition, we also like to identify several cities or slaughter houses that produce the highest quality of beef to validate whether best places for Hanwoo in our analysis are consistent with cities constantly recommended from Google query and many Koreans. Then we calibrate a machine learning model to identify cattle that are most likely to produce the highest-grade beef. According to our calibrated decision tree (DT) model, neutralized male Hanwoo with a certain weight range is most likely to produce high grade meat and our DT model performs at least twice better than a random model in terms of correctly identifying positive samples when top 20% and 40 % of cattle were chosen for a prediction task. Finally, we like to explore different types of beef supply chains in the current digital traceability system and profile them based on their distinct geographical coverages and beef consumption patterns.
Downloads
References
Lim, D., Choi, B.H., Cho, Y.M., Chai, H.H., Jang, G.W., Gondro, C., Jeong. Y.H., & Lee, S.H. (2016). Analysis of extended haplotype in Korean cattle (Hanwoo) population. BMB Report, 49(9), 514-519. doi:10.5483/bmbrep.2016.49.9.074.
Lee, S.-H., Park, B.-H., Sharma, A., Dang, C.-G., Lee, S.-S., Choi, T.-J., Choy, Y.-H., Kim, H.-C., Jeon, K.-J., Kim, S.-D., Yeon, S.-H., Park, S.-B., & Kang, H.-S. (2014). Hanwoo cattle: Origin, domestication, breeding strategies and genomic selection. Journal of Animal Science and Technology, 56(2). doi:10.1186/2055-0391-56-2.
Kim, E.H., Cheong, H.S., Bae, J.S., Chun, J.-Y., Park, T.J., Lee, K.K., Yun, Y.M., & Shin, H.D. (2010). Identification of genetic polymorphisms in bovine mtDNA. Journal of Animal Science, 88(8), 2551-2555. doi:10.2527/jas.2009-2235.
Charlebois, S., Latif, N., Ilahi, I., Sarker, B., Music, J., & Vezeau, J. (2024). Digital traceability in agri-food supply chains: A comparative analysis of OECD member countries. Foods, 13(7), 1075. https://doi.org/10.3390/foods13071075.
Yang, C. (2022). Digital contact tracing in the pandemic cities: Problematizing the regime of traceability in South Korea. Big Data & Society, 9(1), https://doi.org/10.1177/20539517221089294.
Hong, E., Lee, S.Y., Jeong, J.Y., Park, J.M., Kim, B.H., Kwon, K., & Chun, H.S. (2017). Modern analytical methods for the detection of food fraud and adulteration by food category. Journal of the Science of Food and Agriculture, 97(12), 3877–3896. https://doi.org/10.1002/jsfa.8364.
Kamruzzaman, M., Makino, Y., & Oshita, S. (2015). Non-invasive analytical technology for the detection of contamination, adulteration, and authenticity of meat, poultry, and fish: A review. Analytica Chimica Acta, 853, 19-29. https://doi.org/10.1016/j.aca.2014.08.043.
Euro Meat News. (2023). Retrieved from: https://www.euromeatnews.com/Article-Blockchain-beef-traceability-to-be-launched-in-South-Korea/2240 on January, 2025.
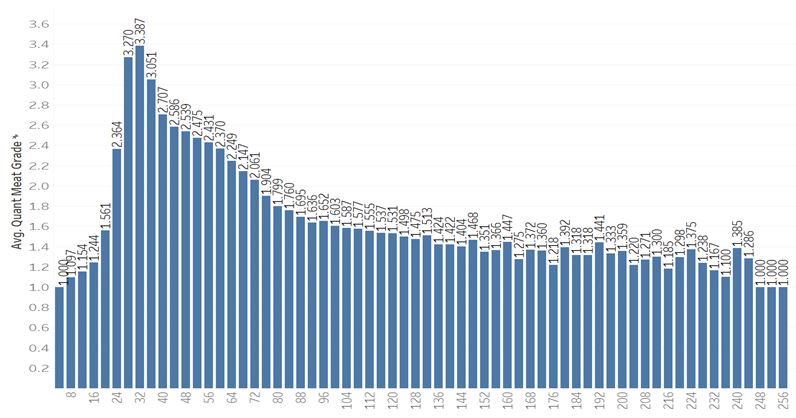
Published
How to Cite
Issue
Section
ARK
License
Copyright (c) 2025 Yong Seog Kim, Hong Nam Nguyen

This work is licensed under a Creative Commons Attribution 4.0 International License.
Research Articles in 'Applied Science and Engineering Journal for Advanced Research' are Open Access articles published under the Creative Commons CC BY License Creative Commons Attribution 4.0 International License http://creativecommons.org/licenses/by/4.0/. This license allows you to share – copy and redistribute the material in any medium or format. Adapt – remix, transform, and build upon the material for any purpose, even commercially.